Accelerating medical research, increasing public safety, building smart cities and continually improving the services used by citizens every day are just a few examples of the benefits that artificial intelligence (AI) can deliver in the public sector, writes Ian Ryan.
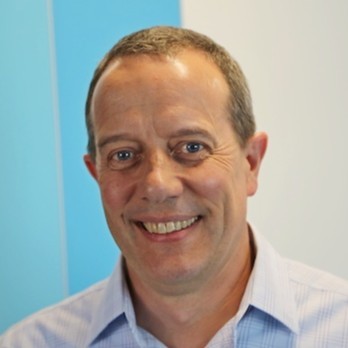
Yet compared with many private sector industries, it’s fair to say that public sector adoption of AI technology has been more measured. Governments and other public sector organisations face a number of significant challenges, from the availability of skills and investment funding, to demonstrating value and ensuring transparency about how decisions are made.
These challenges are reflected in the SAP Institute for Digital Government’s latest report – Building Explainability into Public Sector Artificial Intelligence – developed in partnership with the University of Queensland. While 80 per cent of public sector organisations are actively working towards data-driven transformation, fewer than 15 per cent have progressed beyond prototypes.
In order to drive greater uptake, the public sector needs to develop best practice frameworks and solutions for the development and use of AI systems that are accurate, robust, and scalable, but also reliable, fair, and transparent.
When building AI systems to meet these high levels of expectation, it’s vital that public sector workers are able to understand how these systems generate decisions and explain how this impacts results. This is known as AI explainability.
How AI explainability will help
An AI model sits at the heart of any AI technology. This is a data-based algorithm that’s trained to mimic human decision-making processes. The models are an abstract representation of some portion of reality, designed to predict real-world scenarios.
These models contain inevitable gaps in understanding and performance, all of which need to be addressed and managed. For example, users can’t fully comprehend the model logic, which can inhibit AI trust and acceptance. There are also concerns around inconsistencies between user understanding and reality, potentially creating bias and further reducing adoption in the public sector.
Explainability is helping stakeholders to understand the ‘thinking’ behind AI decisions. The process maps and explains how AI predictions and recommendations align to stakeholder perspectives and perceived outcomes, ensuring the intended value is delivered.
AI explainability helps employees work with AI processes more effectively, especially during the AI training processes, while the systems become increasingly more accurate and results become more meaningful. This enables public sector organisations to better meet the needs of citizens and employees.
Making explainability work
AI explainability is important for any industry, but with the additional need for public sector organisations to demonstrate high levels of transparency and accountability, explainability has a particularly critical role in driving adoption and outcomes. The public sector should look to incorporate the following guidelines on AI projects:
- Simplify explanations and involve stakeholders in model development – It’s critical to consider the knowledge, values and perspectives of users when building an AI model. Training good AI models requires input from multiple stakeholders, including subject matter experts, executives and citizens. Move away from technical discussions and use relatable explanations of the decisions, actions, and mechanics relevant to these stakeholders, encouraging engagement and reducing resistance.
- Ensure user-friendly application interfaces – When a model’s complexity is mirrored by its technical application, it can be challenging for non-technical stakeholders to understand and use the tool. Seamless integration into existing workflows, products and services is just as vital. Provide simple and clear interfaces with visual aids to get the most from AI-augmented decision-making processes.
- Educate and empower frontline staff to explore AI and override errors – Humans should always remain in the decision-loop of any AI system. Those who are continuously informed with explanations will be more likely to integrate the AI into their everyday tasks, thereby maximising the value of the system, and providing additional learning opportunities for the AI.
- Plan for an iterative process when considering adoption – AI technologies are still maturing, so public sector organisations should take the process one step at a time, refining AI systems and models as revisions are needed. Explanations are critical in understanding and supporting these decisions to ensure the best outcomes.
Looking ahead
AI has great potential to play a part in Australian’s recovery from the ongoing impact of the COVID-19 pandemic. However, it’s important that AI projects are approached with the broader context and organisation in mind, rather than as a niche project within the domain of the IT department.
Quality data and human expertise are essential for any organisation looking to use AI technology. AI explainability and appropriate training will ensure that employees are fully engaged in the process and drive maximum value from the investment.
*Ian Ryan is Global Head of SAP’s Institute for Digital Government
Some interesting aspects requiring more research and support the Higher Education establishments. The expertise, knowledge, academic thought processes and supportive professionals have much to contribute and would benefit from further collaboration and engagement.