Automation can help governments process the massive amounts of data being produced, potentially leading to better infrastructure and public transport, writes Ridhav Mahajan.
Big data presents both big opportunities and big challenges. As Australia’s population increases and our cities become more interconnected, a massive amount of data is being produced.
Harnessing this data could lead to better infrastructure, public transport timetables, traffic optimisation and security. There’s just one issue: processing it all.
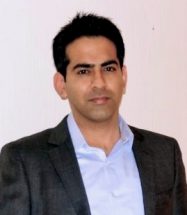
According to recent insights from Deloitte, almost 80 per cent of data scientists’ work can be fully or partially automated, freeing them up to work on higher-value tasks.
Automation could relieve data scientists in government of time consuming tasks like data wrangling, pre-processing and normalising data or filling in missing values.
The challenges of big data
The public sector is starting to use data and analytics to drive value for citizens, but still faces many challenges such as quality and accessibility of data and talent shortages.
Because of this, artificial intelligence (AI) can help with the heavy lifting that data scientists are burdened with. Although AI is arguably a minimal viable product currently, governments should not pass up its potential.
The enormity of big data that the public sector is exposed to is similar to what the private sector experiences. Yet the private sector has been using machine learning (ML) and AI for years to streamline data processes, trialing and incubating the technology. In fact, almost nine in 10 Australian private business leaders have implemented AI into their organisations, according to an Infosys study.
Which raises the question – why doesn’t the public sector follow suit and invest in ways to automate this data?
Time and cost saving are among the benefits ML and AI could bring to the public sector. According to insights from KPMG, the public sector requires funding of $40 billion just to maintain operational functionality of Australia’s $1.7 trillion public sector asset base.
This capital spend, however, does not maintain the status quo if there is growth in the population. Investing in automation can save time on data processes, lowering costs and helping mitigate the risks of budget blowouts.
Examples overseas
There are examples in the US of how utilising AI can drive effective data operations in government.
To improve the security of US computer networks, MapR’s converged data platform enabled the US Department of Homeland Security to build ML models that can detect and identify unauthorised attempts and separate abnormal from regular activities. Removing this process from data personnel freed them up to perform higher value tasks.
An example of where AI could benefit the Australian public sector is in the growing populations in cities like Sydney, Melbourne and Brisbane. With around one million people being added to the population every two to three years, public infrastructure should be top priority for governments.
However, they need the ability to analyse traffic flow data on different roads or in different parts of the city. This means that potential traffic problems in dense urban areas can be prevented by adjusting public transportation routes in real time with the ease of automation.
If the Australian public sector does not adopt automation in these areas it risks being left behind as the rest of the world’s governments digitally transform. By learning from the private sector, governments can make informed-decisions about how and where they should deploy AI.
Ridhav Mahajan is solutions architect ANZ at MapR Technologies.
Comment below to have your say on this story.
If you have a news story or tip-off, get in touch at editorial@governmentnews.com.au.
Sign up to the Government News newsletter.
Leave a Reply